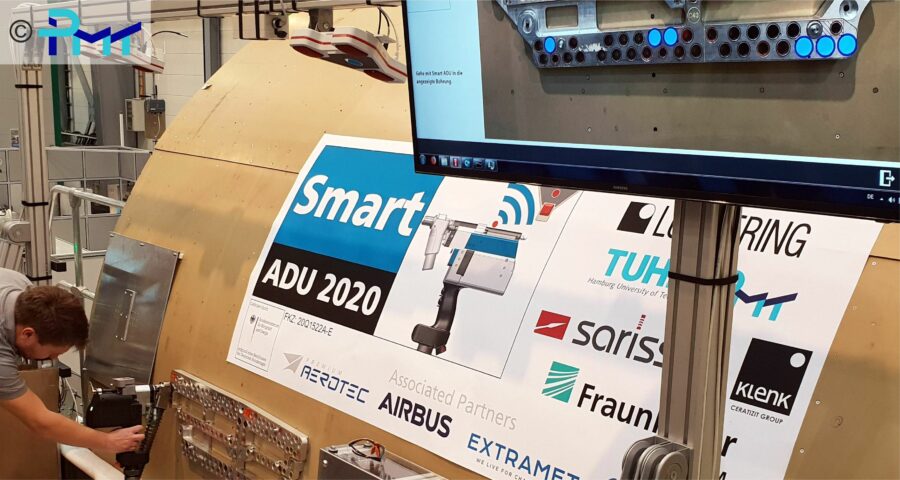
Hamburg, February 24, 2021 – Around 150 million rivets are set every year in European aircraft construction – two thirds of which are processed by employees using lightweight machines. It is obvious that nothing must go wrong here. Quality control is correspondingly complex and expensive. This is where the work of Samuel Bender from the Institute of Production Management and Technology (IPMT) at the Technical University of Hamburg comes in, who has developed a process that further automates the inspection of rivet holes – with the help of artificial intelligence. “If operators are told before or during the drilling process that an error has been detected or that values are outside expectations, they can abort the process or carry out a check directly afterwards,” explains Prof. Wolfgang Hintze from WGP (Wissenschaftliche Gesellschaft für Produktionstechnik) and head of production technology at IPMT Hamburg. “The process can not only change aircraft construction, but also other industries, such as automotive or shipbuilding, in which components are assembled using riveted or screwed joints, and for which holes have to be drilled.” For his bachelor’s thesis on intelligent drilling machines, Bender received first place in the VDMA’s “Digitalization in Mechanical Engineering” young talent award for practical solutions.
In a single aircraft, hundreds of thousands of rivets are set, each requiring a rivet hole. To a large extent, this cannot happen automatically because the current industrial robots are too large, heavy, inflexible or unsuitable for other reasons. But with the semi-automatic drills that have to be positioned by hand by humans, it can happen, for example, that the latter is not clamped properly or, in general, that the tool is already showing signs of wear. In aviation, however, very high safety requirements prevail. “The permissible ridge height on the clamping surface of rivets, for example, is very tightly dimensioned,” explains Hintze, “it is less than a tenth of a millimeter – otherwise the rivet could loosen in flight.” If the value is exceeded, reworking is required. The effort required for inspection is correspondingly high, with a very large number of spot checks and reworking of holes.
An adaptive drilling machine
Operators place the semi-automatic machines on drilling templates that allow precise positioning. The templates were previously attached to the aircraft fuselage. “The actual drilling process is then taken over by the machine,” Samuel Bender specifies. “To do this, it regulates the speed and feed over the entire feed path, as well as other functions such as lubrication and chip extraction. It behaves like a small machine tool.” Bender wanted to make these manufacturing processes predictable and used artificial intelligence methods, in this case machine learning (ML).
At the IPMT of the TU Hamburg, he developed several methods in cooperation with Johannes Lübbering GmbH from Herzebrock-Clarholz, which evaluate various sensor data from the drilling machine prototype manufactured by Lübbering. Among other things, the electrical currents of the two electric motors are recorded. In accordance with the machine learning methodology, the first task was to collect and characterize the signal progressions under known drilling conditions. The machine learns typical progressions of an optimal borehole and compares them with the one currently being drilled. If the signals deviate during the current production process, the machine notices this and reports it. “The drilling machine can reliably predict whether, for example, the correct drilling diameter was used, the expected material combination is present, or the lubricant is active,” Bender explains. Exceeding the diameter tolerance or the burr height, for example, is automatically detected. A prerequisite, however, is that the ML models have previously been supplied with the position-dependent data. This includes, for example, the existing material combination – in aircraft construction, layer packages of aluminum, CFRP and titanium – the thickness of the materials and the necessary engine speed.
Concrete error messages
Thanks to the new process, assembly workers will in future receive not just a general error message, but specific warnings such as “incorrect drilling position”, “attention, lubricant failed” or “tool worn”. In this way, they can avoid the problem or rectify it directly – in other words, replace the tool at an early stage or take other remedial action. “With the help of artificial intelligence, we can already record the required quality during machining. We can thus greatly reduce the very time-consuming and cost-intensive inspection to a few random samples and only have to follow up on detected irregularities,” explains Hintze.
In general, the ML method can be used wherever people or machines perform repetitive but varied machining, assembly or inspection processes. For example, the assembly of safety-critical components or even vehicles can be monitored. Intelligent machines also prevent incipient failures because they detect wear and tear on tools and machine tools at an early stage. In other words, there is an enormous range of possible applications across all industries – always assuming that sufficiently validated and meaningful data is available to train the systems.